When Will Infrastructure Companies See Gains from Generative AI?
- Written by Ed Anuff, Chief Product Officer, DataStax
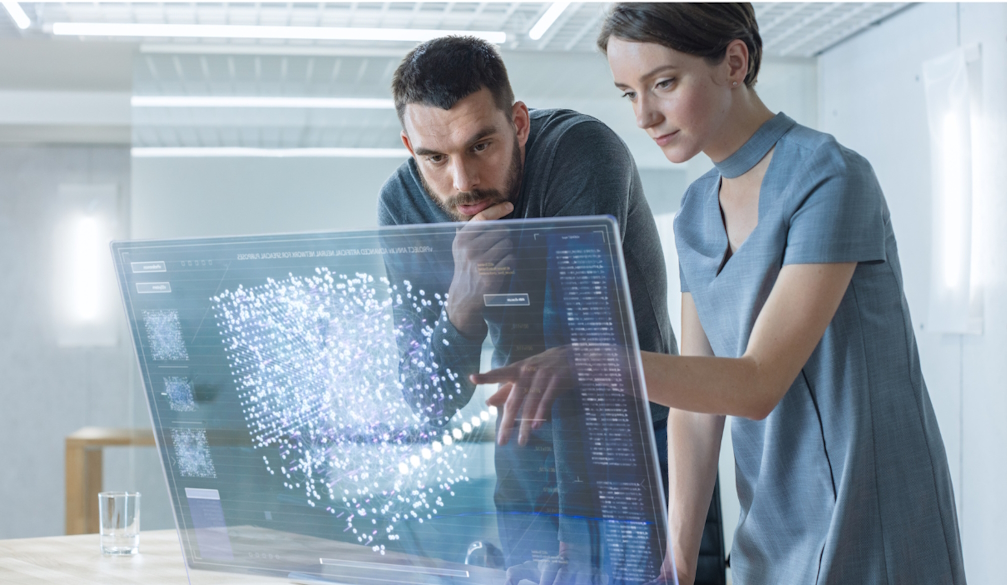
A lot of questions are swirling about the state of generative AI right now. How far along are companies with their bespoke GenAI efforts? Are organisations actually building AI applications using their own proprietary data in ways that move the needle? What kind of architecture is required?
With these questions being asked daily, it’s important to clarify the state of affairs in the GenAI marketplace across three areas: what the market wants to see, fears about what might happen, and what will happen (and how) in 2025.
Three factors affecting the GenAI marketplace
What Does Everyone Want — and What Are They Afraid of?
Since, GenAI exploded on the scene in late 2022 with the release of Chat GPT which showed how powerful and accessible this kind of technology could be, the excitement about the potential upside of AI was everywhere. GenAI was going to be infused into every application at every enterprise. Investors envisioned a hockey-stick-like growth curve for companies that provide the infrastructure to support GenAI.
The cynics, on the other hand, envisioned a dystopian AI future that’s a cross between “Westworld” and “Black Mirror.” Others warn of an AI bubble or something similar to the crypto buzz.
The big difference between GenAI and crypto is the fact that there are many, many real use cases for the former, across organisations and industries. In crypto, there was one strong use case: financial transactions between untrusted parties which is something the mainstream isn’t quite as interested in.
The main issue right now is how businesses can ensure that language models don’t return inaccurate responses by hallucinating.
Where Are We Now, and Where Are We Going?
Last year there were a lot of proof-of-concept GenAI projects: apps to demonstrate to a company’s leadership what’s possible. Very few companies have moved beyond that to build applications that are in full production, meaning that the organisation has an AI application that is being used by customers or employees in a non-prototype way.
The hype cycle
At the moment, we are not as far from reaching the “plateau of productivity” as some may think. Organisations have been struggling with trusting models’ output and they are still grappling with how to produce relevant and accurate large language model (LLM) responses by reducing hallucinations.
RAG (retrieval augmented generation), is being employed to help solve this problem. RAG provides models with additional data or context in real-time from other sources, most often a database that can store vectors. Built on organisations’ most valuable asset: their own data, this technology advancement is key to developing domain-specific, bespoke GenAI applications.
While RAG has emerged as the de facto method for getting enterprise context into GenAI applications, fine-tuning is also mentioned when a pre-trained model is trained further on a subset of data. There are times when this method can be useful, but RAG is the right choice if there’s any concern for privacy, security, or speed.
Regardless of how context is added to the application, the big question in many investors mind is, when will companies start to make money from GenAI apps?
Most of the enterprises are consumption-based businesses. Many are now supporting the experiments, proofs of concept (POCs), and niche apps that their customers have built; those don’t do much in the way of consumption.
This is starting to change as major AI applications start to go from POC into true production. This is predicted to happen in a significant way by the end of 2024 and will come to fruition throughout 2025, starting in two places.
First, it’s taking hold in retail, you will see widespread adoption in the “AI intranet” area: Chat with PDF, knowledge bases and internal call centres.
With the consumption that these kinds of apps drive, companies like Microsoft, Google, and even Oracle are starting to report results from AI. Outside of the realm of hyperscalers, other AI infrastructure companies will likely start to highlight lifts in the earnings reports they release in the first quarter of 2025.
The Path to Production for GenAI Applications
The groundwork has already been laid for consumption-based AI infrastructure companies. There are strong, commercial proof points that show what’s possible for a large base of domain-specific, bespoke applications. From the creative AI apps — Midjourney, Adobe Firefly, and other image generators, for example — to knowledge apps like GitHub, Copilot, Glean, and others, these applications have enjoyed great adoption and have driven significant productivity gains.
Progress on bespoke apps is most advanced in industries and use cases that need to facilitate delivering knowledge to the point of interaction. The knowledge will come from their own data, using off-the-shelf models (either open source or proprietary), RAG, and the cloud provider of their choice.
Three elements are required for enterprises to build bespoke GenAI apps that are ready for the rigors of functioning at the production scale: smart context, relevance, and scalability.
Smart Context
Proprietary data is used to generate useful, relevant, and accurate responses in GenAI applications.
Applications take user input in the shape of all kinds of data and feed it all into an embedding engine, which essentially derives meaning from the data, retrieves information from a vector database using RAG, and builds the “smart context” that the LLM can use to generate a contextualised, hallucination-free response that’s presented to the user in real-time.
Relevance
This isn’t a topic you hear much about at operational database companies. But in the field of AI and vector databases, relevance is a mix of recall and precision that’s critical to producing useful, accurate, non-hallucinatory responses.
Unlike traditional database operations, vector databases enable semantic or similarity search, which is non-deterministic in nature. Because of this, the results returned for the same query can be different depending on the context and how the search process is executed. This is where accuracy and relevance play a key role in how the vector database operates in real-world applications. Natural interaction requires that the results returned on a similarity search are accurate AND relevant to the requested query.
Scalability
GenAI apps that go beyond POCs and into production require high throughput. Throughput essentially is the amount of data that can be stored, accessed, or retrieved in a given amount of time. High throughput is critical to delivering real-time, interactive, data-intensive features at scale; writes often involve billions of vectors from multiple sources, and GenAI applications can generate massive amounts of requests per second.
Wrapping Up
As with earlier waves of technology innovation, GenAI is following an established pattern, and all signs point to it moving even faster than previous tech revolutions. If you cut through all the negative and positive hype about it, it’s clear that promising progress is being made by companies working to move their POC GenAI apps to production.
And companies like DataStax that provide the scalable, easy-to-build-on foundations for these apps will start seeing the benefits of their customers’ consumption sooner than some might think.