A search engine researcher explains the promise and peril of letting ChatGPT and its cousins search the web for you
- Written by Chirag Shah, Professor of Information Science, University of Washington
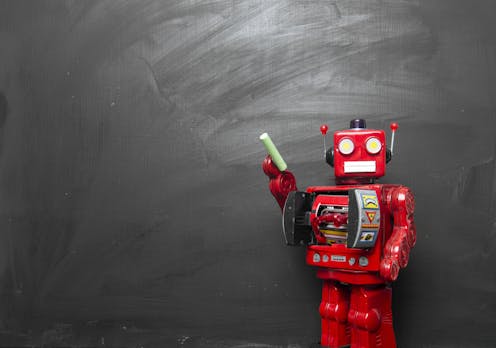
The prominent model of information access before search engines became the norm – librarians and subject or search experts providing relevant information – was interactive, personalized, transparent and authoritative. Search engines are the primary way most people access information today, but entering a few keywords and getting a list of results ranked by some unknown function is not ideal.
A new generation of artificial intelligence-based information access systems, which includes Microsoft’s Bing/ChatGPT[1], Google/Bard[2] and Meta/LLaMA[3], is upending the traditional search engine mode of search input and output. These systems are able to take full sentences and even paragraphs as input and generate personalized natural language responses.
At first glance, this might seem like the best of both worlds: personable and custom answers combined with the breadth and depth of knowledge on the internet. But as a researcher who studies the search and recommendation systems[4], I believe the picture is mixed at best.
AI systems like ChatGPT and Bard are built on large language models. A language model is a machine-learning technique that uses a large body of available texts, such as Wikipedia and PubMed articles, to learn patterns. In simple terms, these models figure out what word is likely to come next, given a set of words or a phrase. In doing so, they are able to generate sentences, paragraphs and even pages[5] that correspond to a query from a user. On March 14, 2023, OpenAI announced the next generation of the technology, GPT-4, which works with both text and image input[6], and Microsoft announced that its conversational Bing is based on GPT-4[7].
‘60 Minutes’ looked at the good and the bad of ChatGPT.Thanks to the training on large bodies of text, fine-tuning and other machine learning-based methods, this type of information retrieval technique works quite effectively. The large language model-based systems generate personalized responses to fulfill information queries. People have found the results so impressive that ChatGPT reached 100 million users in one third of the time it took TikTok to get to that milestone. People have used it to not only find answers but to generate diagnoses[8], create dieting plans[9] and make investment recommendations[10].
Opacity and ‘hallucinations’
However, there are plenty of downsides. First, consider what is at the heart of a large language model – a mechanism through which it connects the words and presumably their meanings. This produces an output that often seems like an intelligent response, but large language model systems are known to produce almost parroted statements[11] without a real understanding. So, while the generated output from such systems might seem smart, it is merely a reflection of underlying patterns of words the AI has found in an appropriate context.
This limitation makes large language model systems susceptible to making up or “hallucinating” answers[12]. The systems are also not smart enough to understand the incorrect premise of a question and answer faulty questions anyway. For example, when asked which U.S. president’s face is on the $100 bill, ChatGPT answers Benjamin Franklin without realizing that Franklin was never president and that the premise that the $100 bill has a picture of a U.S. president is incorrect.
The problem is that even when these systems are wrong only 10% of the time, you don’t know which 10%. People also don’t have the ability to quickly validate the systems’ responses. That’s because these systems lack transparency – they don’t reveal what data they are trained on, what sources they have used to come up with answers or how those responses are generated.
For example, you could ask ChatGPT to write a technical report with citations. But often it makes up these citations[13] – “hallucinating” the titles of scholarly papers as well as the authors. The systems also don’t validate the accuracy of their responses. This leaves the validation up to the user, and users may not have the motivation or skills to do so or even recognize the need to check an AI’s responses.
Stealing content – and traffic
While lack of transparency can be harmful to the users, it is also unfair to the authors, artists and creators of the original content from whom the systems have learned, because the systems do not reveal their sources or provide sufficient attribution. In most cases, creators are not compensated or credited[14] or given the opportunity to give their consent.
There is an economic angle to this as well. In a typical search engine environment, the results are shown with the links to the sources. This not only allows the user to verify the answers and provides the attributions to those sources, it also generates traffic for those sites[15]. Many of these sources rely on this traffic for their revenue. Because the large language model systems produce direct answers but not the sources they drew from, I believe that those sites are likely to see their revenue streams diminish.
Taking away learning and serendipity
Finally, this new way of accessing information also can disempower people and takes away their chance to learn. A typical search process allows users to explore the range of possibilities for their information needs, often triggering them to adjust what they’re looking for. It also affords them an opportunity to learn[16] what is out there and how various pieces of information connect to accomplish their tasks. And it allows for accidental encounters or serendipity[17].
These are very important aspects of search, but when a system produces the results without showing its sources or guiding the user through a process, it robs them of these possibilities.
Large language models are a great leap forward for information access, providing people with a way to have natural language-based interactions, produce personalized responses and discover answers and patterns that are often difficult for an average user to come up with. But they have severe limitations due to the way they learn and construct responses. Their answers may be wrong, toxic or biased[18].
While other information access systems can suffer from these issues, too, large language model AI systems also lack transparency. Worse, their natural language responses can help fuel a false sense of trust and authoritativeness[19] that can be dangerous for uninformed users.
References
- ^ Bing/ChatGPT (www.bing.com)
- ^ Google/Bard (blog.google)
- ^ Meta/LLaMA (ai.facebook.com)
- ^ studies the search and recommendation systems (scholar.google.com)
- ^ generate sentences, paragraphs and even pages (dx.doi.org)
- ^ works with both text and image input (openai.com)
- ^ conversational Bing is based on GPT-4 (techcrunch.com)
- ^ generate diagnoses (www.statnews.com)
- ^ create dieting plans (www.cnet.com)
- ^ make investment recommendations (www.upstart.com)
- ^ known to produce almost parroted statements (doi.org)
- ^ “hallucinating” answers (www.datanami.com)
- ^ makes up these citations (blogs.library.duke.edu)
- ^ not compensated or credited (medium.com)
- ^ generates traffic for those sites (searchengineland.com)
- ^ opportunity to learn (doi.org)
- ^ accidental encounters or serendipity (doi.org)
- ^ wrong, toxic or biased (www.fastcompany.com)
- ^ false sense of trust and authoritativeness (nymag.com)