AI is inherently ageist. That’s not just unethical – it can be costly for workers and businesses
- Written by Sajia Ferdous, Lecturer in Organisational Behaviour, Queen's Business School, Queen's University Belfast
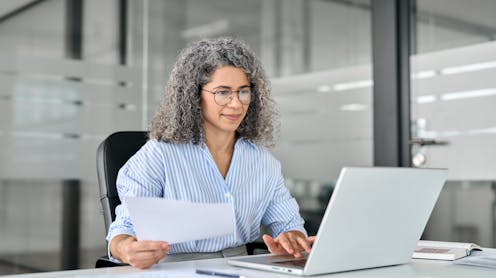
The world is facing a “silver tsunami” – an unprecedented ageing of the global workforce. By 2030, more than half of the labour force in many EU countries[1] will be aged 50 or above. Similar trends are emerging across Australia[2], the US[3] and other developed and developing economies[4].
Far from being a burden or representing a crisis, the ageing workforce is a valuable resource – offering a so-called “silver dividend”[5]. Older workers often offer experience, stability and institutional memory. Yet, in the rush to embrace artificial intelligence (AI), older workers can be left behind[6].
One common misconception is that older people are reluctant[7] to adopt technology or cannot catch up. But this is far from the truth. It oversimplifies the complexity of their abilities, participation and interests in the digital environments.
There are much deeper issues and structural barriers at play. These include access and opportunity – including a lack of targeted training[8]. Right now, AI training tends to be targeted at early or mid-career workers.
Ageism has long shaped hiring, promotion and career development. Although age has become a protected characteristic[17] in UK law, ageist norms and practices persist in many not-so-subtle forms.
Ageism can affect both young and old, but when it comes to technology, the impact is overwhelmingly skewed against older people.
So-called algorithmic ageism[18] in AI systems – exclusion based on automation rather than human decision-making – often exacerbates ageist biases.
Hiring algorithms[19] often end up favouring younger employees. And digital interfaces that assume tech fluency are another example of exclusionary designs. Graduation dates, employment gaps, and even the language used in CVs can become proxies for age and filter out experienced candidates without any human review.
Tech industry workers are overwhelmingly young[20]. Homogenous thinking breeds blind spots, so products work brilliantly for younger people. But they can end up alienating other age groups.
This creates an artificial “grey digital divide”[21], shaped less by ability and more by gaps in support, training and inclusion. If older workers are not integrated into the AI revolution, there is a risk of creating a divided workforce. One part will be confident with tech, data-driven and AI-enabled, while the other will remain isolated, underutilised and potentially displaced.
It’s vital to move beyond the idea of being “age-inclusive”, which frames older people as “others” who need special adjustments. Instead, the goal should be age-neutral designs.
AI designers should recognise that while age is relevant in specific contexts – such as restricted content like pornography – it should not be used as a proxy in training data, where it can lead to bias in the algorithm. In this way, design would be age-neutral rather than ageless.
Designers should also ensure that platforms are accessible for users of all ages.
The stakes are high. It is also not just about economics, but fairness, sustainability and wellbeing.
At the policy level in the UK, there is still a huge void. Last year, House of Commons research[22] highlighted that workforce strategies rarely distinguish the specific digital and technological training needs of older workers. This underscores how ageing people are treated as an afterthought.
A few forward-thinking companies[23] have backed mid- and late-career training programmes. In Singapore, the government’s Skillsfuture programme[24] has adopted a more agile, age-flexible approach. However, these are still isolated examples.
Retraining cannot be generic. Beyond basic digital literacy courses, older people need targeted, job-specific advanced training. The psychological framing of retraining is also critical. Older people need to retrain or reskill not for just career or personal growth but also to be able to participate more fully in the workforce.
It’s also key for reducing pressure on social welfare systems and mitigating skill shortages. What’s more, involving older workers in this way supports the transfer of knowledge between generations, which should benefit everyone in the economy.
Yet, currently, the onus is on the older workers and not organisations and governments.
AI, particularly the generative models that can create text, images and other media, is known for producing outputs that appear plausible but are sometimes incorrect or misleading[25]. The people best placed to identify these errors are those with deep domain knowledge – something that is built over decades of experience.
This is not a counterargument to digital transformation or adoption of AI. Rather, it highlights that integrating older people into digital designs, training and access should be a strategic imperative. AI cannot replace human judgment yet – it should be designed to augment it[26].
If companies, policies and societies exclude older workers from AI transformation processes, they are essentially removing the critical layer of human oversight that keeps AI outputs reliable, ethical and safe to use. An age-neutral approach will be key to addressing this.
Piecemeal efforts and slow responses could cause the irreversible loss of a generation of experience, talent and expertise. What workers and businesses need now are systems, policies and tools that are, from the outset, usable and accessible for people of all ages.
References
- ^ many EU countries (www.ilo.org)
- ^ Australia (cepar.edu.au)
- ^ the US (www.pewresearch.org)
- ^ developing economies (www.pbs.org)
- ^ “silver dividend” (www.adb.org)
- ^ left behind (www.weforum.org)
- ^ reluctant (www.ons.gov.uk)
- ^ lack of targeted training (www.forbes.com)
- ^ Sign up to our daily newsletter (theconversation.com)
- ^ Join The Conversation for free today (theconversation.com)
- ^ exclusionary (www.pewresearch.org)
- ^ Data (www.pewresearch.org)
- ^ Voice assistants (pmc.ncbi.nlm.nih.gov)
- ^ fintech apps (www.theguardian.com)
- ^ socio-demographic factors (www.ageing.ox.ac.uk)
- ^ Andrey_Popov/Shutterstock (www.shutterstock.com)
- ^ protected characteristic (www.equalityhumanrights.com)
- ^ algorithmic ageism (www.ageing.ox.ac.uk)
- ^ Hiring algorithms (www.ifow.org)
- ^ overwhelmingly young (www.linkedin.com)
- ^ “grey digital divide” (pubmed.ncbi.nlm.nih.gov)
- ^ House of Commons research (committees.parliament.uk)
- ^ forward-thinking companies (oats.org)
- ^ Skillsfuture programme (www.skillsfuture.gov.sg)
- ^ incorrect or misleading (mitsloanedtech.mit.edu)
- ^ augment it (hbr.org)